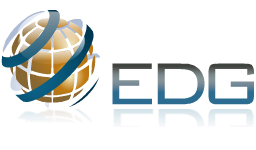
New prediction models can allow utilities to rely more heavily on wind and save millions.
The forecasts, developed by the National Center for Atmospheric Research (NCAR) in Boulder, Colorado, could help address an increasing problem with wind power: how to integrate this intermittent resource into the power grid. NCAR is also developing improved models that could help forecast power from the sun.
“Having an accurate forecast allows us to bring on more renewable energy,” says Drake Bartlett, who is responsible for integrating renewables into Xcel’s grid. Utilities decide a day in advance which power plants will be operating on a given day. Inaccurate wind-power forecasts cause problems with this scheduling in two main ways. First, they force utilities to schedule backup power plants. These run inefficiently at low power, waiting to ramp up their production if less wind power is available than predicted. They waste fuel and are expensive to operate.
Second, bad forecasts make it difficult for utilities to justify shutting down baseload power plants, even if there might be more than enough wind to make them unnecessary. These plants—often coal or combined-cycle natural-gas plants—are expensive and time-consuming to shut down and restart. If they’re shut down and wind power is lower than expected, the utility has to use more expensive power from faster-responding plants or turn to the high-priced spot market. If, on the other hand, the utility leaves the baseload plants on, it may have to curtail wind power, perhaps by telling wind farms to turn off some turbines. In that case, the utility loses money two ways. It has to pay for the fuel to run the baseload plants, even though it didn’t really need power from them. And under its contract with wind farm operators, it still has to pay for the wind power it isn’t using.
The old forecasts allowed these scenarios to arise often. On average, predictions differed from actual power output by 20 percent, and sometimes they were as much as 50 percent off. The new forecasts decrease error by 30 to 40 percent, giving Xcel the confidence to reduce the number of backup plants online, Bartlett says. This has saved the utility $22 million in fuel. It hasn’t calculated the additional cost savings that have come from being able to avoid the spot market.
The new forecasts are also accurate enough to support shutting down baseload power. “A few years ago, we didn’t have the confidence to shut off baseload plants,” Bartlett says. “Now, if it’s a long weekend with beautiful weather and lots of wind, we’ll shut down a coal plant. That allows us to integrate more renewable energy.”
NCAR took several steps to make better forecasts. It improved on previous weather prediction models by running them at finer increments of time and space—something that requires added computing power. It combined its models with those from other organizations and with measurements of actual conditions at wind farms to predict wind speeds. Crucially, it then converts these wind speed predictions to estimates of how much power wind farms will produce, something that can differ considerably from what manufacturers claim (see “Better Computer Models Needed for Mega Wind Farms”).
Also, instead of just running a model once, NCAR runs it multiple times. The average result is typically more accurate, says Sue Ellen Haupt, director of the center’s Weather Systems Assessment Program.
Even though the models are run at high resolution, they don’t catch everything. The next step is to focus on better ways to predict two kinds of events—changes in wind speed, and weather that causes ice to form on wind turbine blades.
Fast changes in wind speed can be particularly difficult to deal with on the grid (see “Wind Turbines, Batteries Included, Can Keep Power Supplies Stable”). Icing predictions will also be important; it’s difficult to know just when a storm will create the right conditions to deposit ice on wind turbines, but when ice does form, it can greatly decrease the amount of power a turbine can generate. In the past, forecasts have led Xcel to plan for large amounts of wind, only to have power production drop off unexpectedly when ice formed.
Predicting solar power may be a bigger challenge altogether (see “A Solution to Solar Power Intermittency”). Power output from solar panels can change in seconds, and clouds are among the hardest things to account for in climate models. NCAR is using data from satellites and land-based sensors to improve its cloud predictions, and it’s working to predict how different amounts of sunlight (and other factors like temperature) translate into actual power output from solar panels.